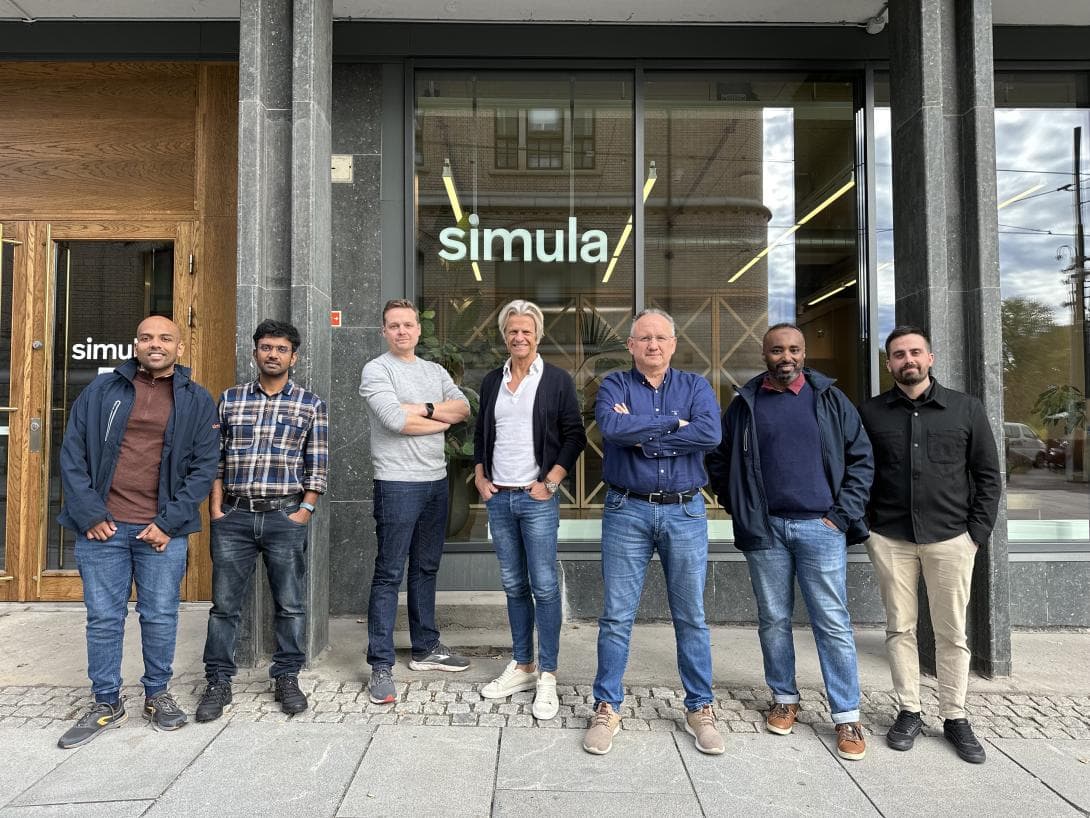
New NFR project: AI-based decentralized controllers for improving efficiency and autonomy of complex networks
Published:
In the DISCO (Distributed Learning and Cooperative Optimization for Multi-agent Autonomous Wireless Access Points) project, a new generation of decentralized data-driven and Machine Learning-based methods will be designed to enable distributed learning and cooperative optimization within complex intelligent network ecosystems.
The DISCO project has received funding from The Research Council of Norway through the program IKTPLUSS. The project has been granted 17.6 million NOK for a four-year duration. DISCO will be led by Chief Research Scientist and Research Professor Baltasar Beferull-Lozano, Head of the Department of Signal and Information Processing for Intelligent Systems (SIGIPRO) at SimulaMet.
The other partners in the project are the University of Oslo (UiO), Nokia Bell Labs, Altibox, Multinett, the Royal Institute of Technology (KTH), and Pompeu Fabra University (UPF).
The project’s central application focus is on large-scale Wi-Fi Access Points (AP) where these methods can enhance their efficiency and autonomy by cooperating together while at the same time minimizing the need for centralized control. However, the theoretical prescriptions and the algorithms designs will also provide insight into developing similar methods for other types of interacting dynamic systems, to learn and perform cooperatively complex tasks in other applications, such as distributed intelligence for teams of robots or windfarms.
Current limitations
Even though Wi-Fi networks are the primary medium for global internet traffic, the current Wi-Fi landscape is characterized by suboptimal performance due to a lack of coordination among multiple interfering Wi-Fi networks. These networks have evolved tremendously, bringing new features not previously present, however, they come together with a huge plethora of configuration parameters, which makes them extremely complex to optimize dynamically.
Current solutions for network resource allocation mainly rely on models and heuristics, lacking a data-driven foundation. These approaches struggle to efficiently explore the vast configuration space across all APs. Additionally, existing machine learning-based solutions assume a centralized controller, which is impractical and can not be assumed for separately owned Wi-Fi networks, in addition to raising a privacy concern.
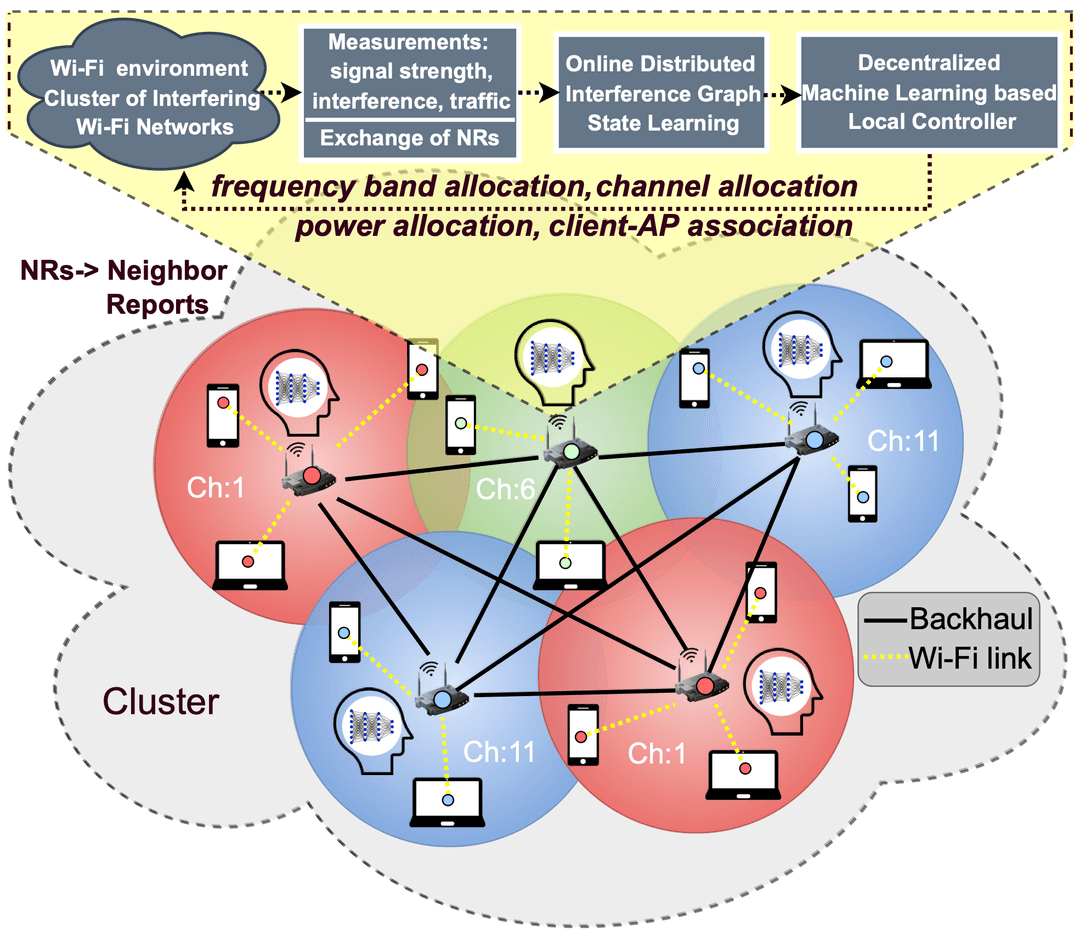
DISCO introduces innovative features
Distributed online machine learning methods: Automated Machine Learning (ML) methods based on online decentralized optimization and graph signal processing, which can efficiently learn and track multi-modal dynamic graphs representing the global state of operation of complex dynamic systems, using various multi-variate and multimodal data measurements. These methods will infer which and how the relevant data variables are affecting each other and will also allow forecasting of the behavior of these networks, as well as missing data imputation and anomaly detection.
Distributed causality-enhanced Multi-Agent Reinforcement Learning (MARL): A fully distributed MARL algorithm for resource allocation, based on learning-based adaptive control, which will exploit a learned state representation of the overall network dynamics. The multiple agents will exchange limited information with each other, in order to perform adaptive sequential control of the various resource allocations. This will be done through in-network cooperation across the APs, without communicating to a cloud center, and achieving a performance in terms of relevant metrics (such as throughput, latency, reliability) that is superior to the currently existing solutions.
To demonstrate the practicality of these solutions, DISCO will implement them as portable containerized software modules and collaborate with prominent national and international stakeholders: Altibox, Multinett, and Bell Labs.
In addition to improving critical performance KPIs, this project will generate additional practical impacts for the related industry and end-users, such as lower CAPEX and OPEX, less energy use environmental footprint, less radiation and improved privacy.