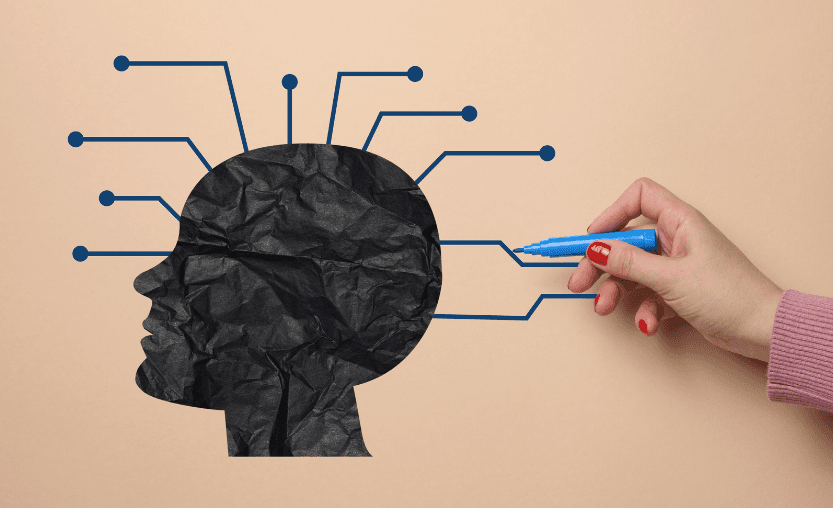
New project: Digitising child and adolescent psychiatry
Published:
In a newly funded project, SimulaMet will contribute to digitise and automate the diagnostic assessment process in Child and Adolescent Psychiatry (CAP) using an AI-based solution.
Mental illness is a leading cause of disability among young people, and many disorders remain undetected. The most common diagnostic method today, face-to-face meetings with patients, provides insufficient information about symptoms and increases the risk of missed diagnoses.
A more effective approach is the structured diagnostic interview. One of the project partners, the Swedish hospital Region Västmanland (RV), has developed digital psychiatric assessment tools supporting this method. These tools, used for patient screening, triage, prioritisation, and diagnosis, have increased patient throughput by 130 percent.
Despite their success, the process of information gathering remains time-consuming. With an escalating number of patients seeking care, there is a need to further reduce this time.
DADAP (Digitizing and Automating the Diagnostic psyciathric Assessment Process)
The 3 year project is coordinated by RISE Research Institutes of Sweden and brings together five partners from Sweden, Norway, Spain and Romania. In addition to RISE and SimulaMet as research partners, three clinical pilots are included, RV, CEMEX and IBIMA-FIMABIS. The project is funded by EU and the Research Council of Norway through The European Partnership on Transforming Health and Care Systems (THCS).
Research Scientist Vajira Thambawita explains how they will improve these tools "the main objective of this project is to make these assessment tools more intelligent by using AI to automate the process, and enhance the capabilities of these tools." Thambawita will lead the work on data infrastructure for this project.
Mirroring the original data
To ensure the privacy of sensitive information gathered during psychiatric evaluations, deep generative models will be used to create synthetic data that mirrors the statistical characteristics of the original data.
“At SimulaMet we have a lot of experience in research with synthetic data generation, and this is why RISE reached out to us to contribute”, says Thambawita.
Researchers in the Department of Holistic Systems at SimulaMet are conducting cutting-edge research on synthetic data creation in the medical domain, and in the application of Large Language Models (LLMs) for generating context-specific textual data. One of their ongoing projects involves training police officers in Norway on interviewing children who have been subject to abuse.
The synthetic data in this project will be generated from standardized questions and answers from care seekers, their relatives, clinically validated assessments, and psychiatric diagnoses.
“We will figure out how to capture the patterns and generate similar patterns based on fake questions and answers to ensure that we have high-quality data for the training and testing of the AI solutions.”
AI-based screening and diagnostic tool
SimulaMet will also contribute to the development of an AI-based screening and diagnostic tool. Trained on the synthetic data, this tool aims to optimise question-asking, leading to more effective diagnosis and treatment selection.
“This will be a tool to support and guide the doctors in the process of asking the most relevant questions, and not to replace the doctors in this process.”
The tool, developed using deep learning techniques, will assist clinicians through a web interface, facilitating early detection and diagnosis, and reducing subjectivity and bias.
Trust in the black box model
Deep learning is an AI method inspired by human brains, it can learn from examples. Using multi-layered neural networks to simulate the complex decision-making power of our brain.
“This domain is often referred to as the black box model. Models trained with deep learning methods, like Open AI’s GPT 4, can have up to a trillion parameters. No one can understand how these are connected to each other and how the model reaches the final output answer.”
This raises the issue of trust. The system has to be explainable and trustworthy for clinicians, ensuring that they can understand and trust its outputs.
“Clinicians in hospitals can’t really understand anything about AI, or how to interpret these outputs. A big part of this project is building an explainable tool that can be trusted, where the process behind the outputs are explained with high confidence.”
By using explainable AI methods, the tool developed will provide descriptions of how the model has come to it’s decisions - what factors are considered and how they are weighed.
Further adaptation
To build on the potential in this project, the consortium is aiming to translate and adapt the instruments to other settings. Automating assessment processes in different healthcare services across countries, languages and cultures.
See the announcement of the project on The European Partnership on Transforming Health and Care Systems (THCS) website.
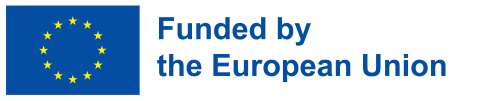
Disclaimer: Funded by the European Union. Views and opinions expressed are however those of the author(s) only and do not necessarily reflect those of the European Union or CINEA. Neither the European Union nor the Granting authority can be held responsible for them.