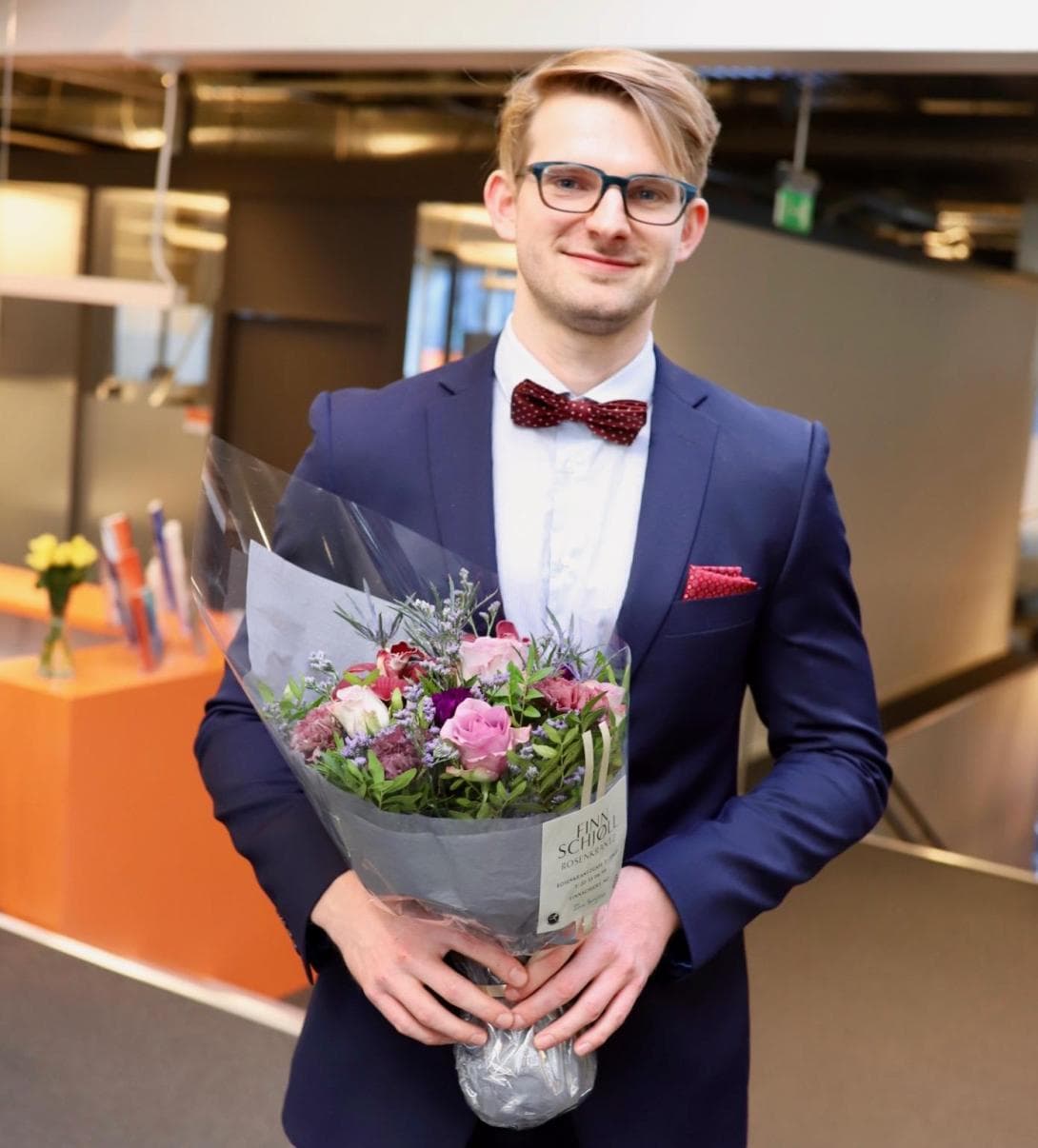
Timo Klock sucessfully defended his PhD
Published:
On Friday, February 28th, Timo Klock successfully defended his PhD thesis, "Model-based function learning in high dimensions." The defense took place at Simula Research Laboratory, Fornebu.
Summary
Successful machine learning systems require a relatively large amount of computing power and large data sets that store high-quality information. While the first demand has been met by the increase in computing power in the past two decades, acquiring sufficiently large data sets is still considered expensive and often represents a bottleneck in practice. This thesis addresses the data bottleneck through model-based machine learning algorithms that lead to computationally efficient algorithms tailored to the data-poor regime, and with rigorously proven performance guarantees.
Before the defense, Timo Klock presented his trial lecture"Regularization of linear inverse problems."
Main research findings
A common task in machine learning centers around identifying functions that describe the relationship between inputs and outputs for a given data set. Although often perceived differently, designing such an algorithm requires knowledge about the data set at hand and a degree of fine-tuning by an experienced data analyst. This is especially true if the available data set is small, and the inputs are highly complex, for instance, because they are embedded in a high-dimensional space. Dealing with such data sets requires the use of model-based machine learning methods that assume the input-output relation can be described in a simplified fashion.
This thesis focuses on models that assume the input-output relation depends on a relatively small number of linear or nonlinear transformations of the original inputs of the data. This includes popular models such as single- and multi-index models, nonlinear generalizations thereof, and certain types of neural networks. We develop computationally, efficient algorithms, and provide performance guarantees through rigorous mathematical analysis. The usability of our methods for practice is ensured by comparisons with state of the art on real-world benchmarks.
Adjudication committee
- Associate Professor Jan Vybiral,Department of Mathematics, Czech Technical University, Czech Republic
- Professor Rima Alaifari,ETH Zurich, Switzerland
- Associate Professor Joakim Sundnes,Department of Informatics, University of Oslo, Norway
Supervisors
- Senior Researcher Valeriya Naumova, Simula Research Laboratory,
- Professor Are Magnus Bruaset, Department of Informatics, UiO / Simula Research Laboratory
Chair of defense
- LecturerDag Frette Langmyhr, Department of Informatics, University of Oslo