Langtangen Seminar
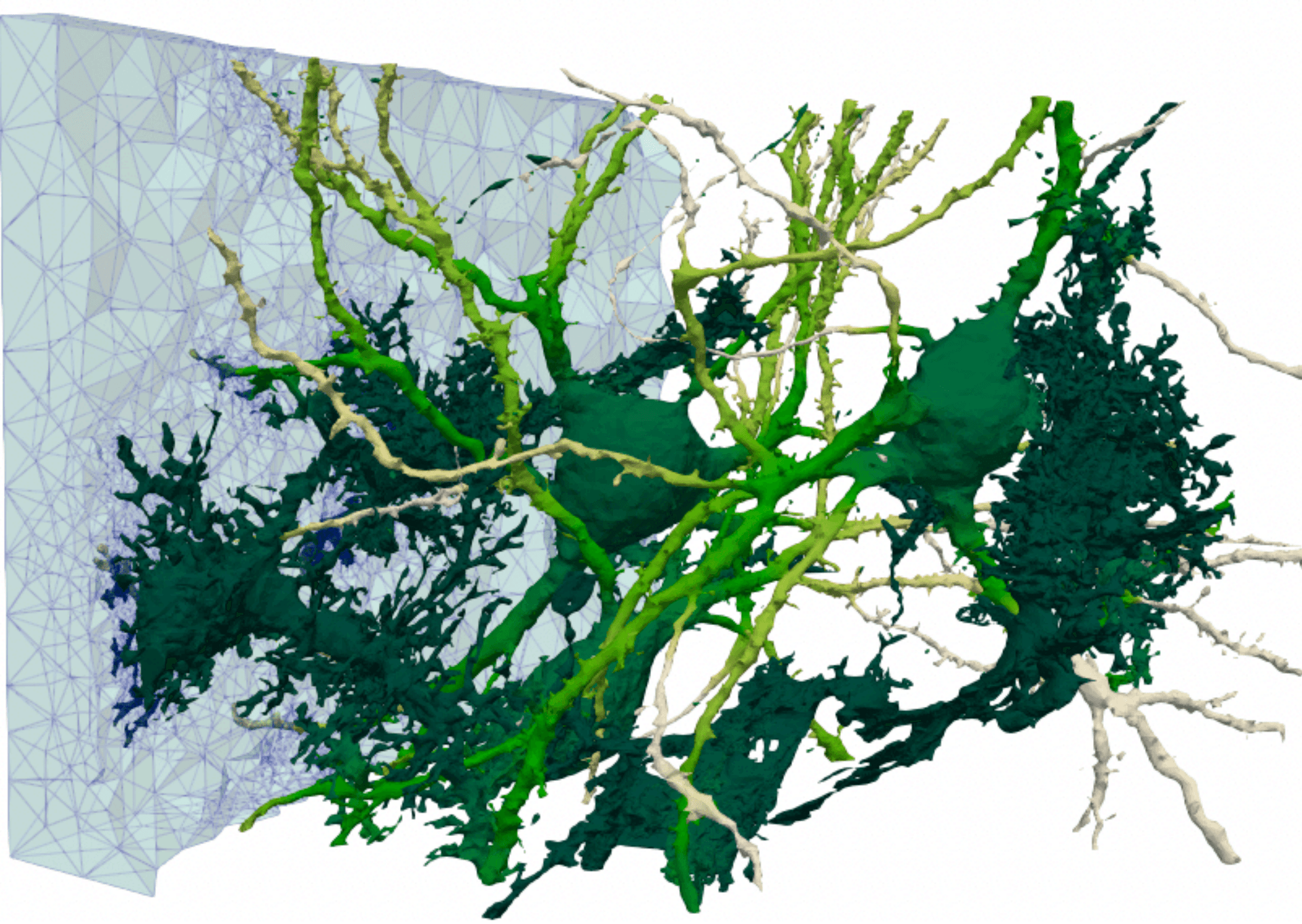
Named in honour of Professor Hans Petter Langtangen, the Langtangen Seminar on Scientific Computing series is part of Simula's continued efforts to be at the forefront of scientific computing. Through this seminar series, we aim to showcase internationally renowned researchers who will not only present their latest, cutting-edge work, but also share their personal insights on the future of the field.
The seminars will be online and viewable via zoom on Tuesday at 15:00 (Central European Time). Some presentations will become available on YouTube on this channel.
Schedule
May 13: Aslak Tveito, Simula Research Laboratory
Upcoming Seminar
- When: Tuesday 13 May 2025 at 15:00
- Where: Join online via Zoom
- Who: Aslak Tveito, Simula Research Laboratory
Computational models of cardiac electrophysiology — from millimeters to micrometers to nanometers
Abstract:
Despite their remarkable success over many decades, numerical methods for approximating PDEs can incur a very high computational cost. This limitation has provided the impetus for the design of fast and accurate Machine Learning/AI based neural PDE surrogates which can learn the PDE solution operator from data.
In this talk, we review some of the latest developments in the field of Neural Operators, which are widely used as an ML paradigm for PDEs and discuss state of the art neural operators based on convolutions or attention. We will discuss graph and transformer based architectures for PDEs on arbitrary domains and conditional Diffusion models for PDEs with chaotic multiscale solutions. Finally, the issue of sam
Computational models of cardiac electrophysiology have seen remarkable progress over the past decades. In the 1980s, whole-heart simulations were considered impossible—the estimated solution time was around 3,000 years. That estimate turned out to be overly pessimistic: by 2011, the problem was solved in five minutes. However, the Bidomain model used in these computations is, by construction, a coarse description of the system. Cardiomyocytes are not explicitly represented; in typical simulations, one computational mesh block covers close to a thousand cells. This highlights a fundamental lack of resolution. At the millimeter scale, the Bidomain model is adequate. But the cell itself operates on the micrometer scale, where a different modeling approach is required.
More recent models resolve individual cardiomyocytes, operating at the micrometer level. This comes at the price of a significantly harder mathematical problem, but with the advantage of enabling detailed representations of single cells. The action potential in each cell is driven by ion channels located at the cell membrane.
Understanding the dynamics near individual ion channels requires yet finer resolution—down to the nanometer scale. At this level, the appropriate mathematical description is the Poisson-Nernst-Planck system. Solving these equations is challenging, but they provide access to the Debye layer and rely almost entirely on physical constants, offering a model closer to basic physics than the approaches mentioned above.
In this talk, I will give a gentle walkthrough of these models, discuss the computational difficulties they pose, and outline some of the physiological questions that can be studied (and in some cases have been studied) using them.
Join the Zoom webinar.
More information
This seminar is organised by Marie E. Rognes & Thomas M. Surowiec.
Join the Zoom webinar.
Past seminars in this series
Learning PDEs
Siddhartha Mishra, Eidgenössische Technische Hochschule Zürich
Abstract:
Despite their remarkable success over many decades, numerical methods for approximating PDEs can incur a very high computational cost. This limitation has provided the impetus for the design of fast and accurate Machine Learning/AI based neural PDE surrogates which can learn the PDE solution operator from data.
In this talk, we review some of the latest developments in the field of Neural Operators, which are widely used as an ML paradigm for PDEs and discuss state of the art neural operators based on convolutions or attention. We will discuss graph and transformer based architectures for PDEs on arbitrary domains and conditional Diffusion models for PDEs with chaotic multiscale solutions. Finally, the issue of sample complexity is addressed by the design of general purpose Foundation models for PDEs.
Mathematical imaging and structure-preserving deep learning
Carola Schönlieb, University of Cambridge
Abstract:
Images are a rich source of beautiful mathematical formalism and analysis.
Associated mathematical problems arise in functional and non-smooth analysis, the theory and numerical analysis of nonlinear partial differential equations, inverse problems, harmonic, stochastic, and statistical analysis, and optimization, just to name a few. Applications of mathematical imaging are profound and arise in biomedicine, material sciences, astronomy, digital humanities, as well as many technological developments such as autonomous driving, facial screening and many more.
In this talk I will discuss my perspective onto mathematical imaging, share my fascination and vision for the subject. I will then zoom into structure-preserving deep learning and its relevance for solving inverse imaging problems.
Enforcing conservation laws and dissipation inequalities via auxiliary variables
Patrick E. Farrell, University of Oxford
Abstract:
We propose a general strategy for enforcing multiple conservation laws and dissipation inequalities in the numerical solution of initial value problems. The key idea is to represent the conservation law or dissipation inequality by means of its associated test function; we introduce auxiliary variables representing the projection onto a discrete test set, and modify the equation to use these new variables. We demonstrate the ideas by generalizing to arbitrary order the energy-dissipating and helicity-tracking scheme of Rebholz for the incompressible Navier–Stokes equations, and by devising a novel time discretization of the compressible Navier–Stokes equations that for the first time conserves mass, momentum, and energy, while probably dissipating entropy.
Modeling neurodegenerative disease
Paola F. Antonietti from Politecnico di Milano
Abstract:
Neurodegenerative diseases (NDs) are complex disorders that mainly affect neurons in the brain and nervous system, resulting in progressive functional decline and structural deterioration. A common pathological hallmark among various NDs is the accumulation of disease-specific misfolded and aggregated proteins, such as amyloid-beta and tau in Alzheimer's disease and α-synuclein in Parkinson's disease. This talk centers on the mathematical and numerical modeling of the fundamental mechanisms that drive and sustain neurodegeneration.
First, we discuss the dynamics of misfolded protein modeling using increasingly complex mathematical models. We develop and analyze high-order discontinuous Galerkin methods on polyhedral grids (PolyDG) to simulate these processes accurately. In the second part of the talk, we present mathematical and computational models of waste clearance mechanisms in the brain, which are critical to the onset and progression of neurodegenerative diseases (NDs). Specifically, we investigate glymphatic and cerebrospinal fluid dynamics and their roles in neurodegenerative processes. Furthermore, we discuss modeling seizure dynamics, focusing on their interplay with pathological protein accumulation. We present numerical simulations based on patient-specific brain geometries reconstructed from clinical imaging data, providing insights into the complex interactions involved in neurodegeneration.
Control and Machine Learning
Enrique Zuazua, Friedrich-Alexander-Universität Erlangen-Nürnberg
Abstract:
Systems control, or cybernetics—a term first coined by Ampère and later popularized by Norbert Wiener—refers to the science of control and communication in animals and machines. The pursuit of this field dates back to antiquity, driven by the desire to create machines that autonomously perform human tasks, thereby enhancing freedom and efficiency.
The objectives of control systems closely parallel those of modern Artificial Intelligence (AI), illustrating both the profound unity within Mathematics and its extraordinary capacity to describe natural phenomena and drive technological innovation.
In this lecture, we will explore the connections between these mathematical disciplines and their broader implications. We will also discuss our recent work addressing two fundamental questions: Why does Machine Learning perform so effectively? And how can data-driven insights be integrated into the classical applied mathematics framework, particularly in the context of Partial Differential Equations (PDE) and numerical methods? This effort is leading us to a new emerging field of PDE+D(ata) in parallel to the development of new Digital Twins technologies.